Stuart F. Quan, M.D.1,2,6
Rohit Budhiraja, M.D.3
Salma Batool-Anwar, M.D., M.P.H.2
Daniel J. Gottlieb, M.D., M.P.H.1,2,4
Phillip Eichling, M.D., M.P.H.7,8
Sanjay Patel, M.D., M.S.1,2
Wei Shen, M.D.6,9
James K. Walsh, Ph.D.5
Clete A. Kushida, M.D., Ph.D.10
1Division of Sleep Medicine, Harvard Medical School, Boston, MA
2Division of Sleep and Circadian Disorders, Brigham and Women’s Hospital, Boston, MA
3Department of Medicine, Tufts University School of Medicine, Boston, MA
4VA Boston Healthcare System, Boston, MA
S5leep Medicine and Research Center, St. Luke's Hospital, Chesterfield, MO
6Arizona Respiratory Center, University of Arizona, Tucson, AZ
7College of Medicine, University of Arizona, Tucson, AZ
8Comprehensive Sleep Solutions, Tucson, AZ
9Southern Arizona VA Health Care System, Tucson, AZ
10Stanford University Sleep Clinic and Center for Human Sleep Research, Redwood City, CA
Abstract
Background and Objectives: Obstructive sleep apnea (OSA) is associated with sleepiness, depression and reduced quality of life. However, it is unclear whether mild OSA has these negative impacts. Using data from the Apnea Positive Pressure Long-term Efficacy Study (APPLES), this study determined whether participants with mild OSA had greater sleepiness, more depressive symptoms and poorer quality of life in comparison to those without OSA.
Methods: 239 persons evaluated for participation in APPLES with a baseline apnea hypopnea index (AHI) < 15 /hour were assigned to 1 of 2 groups: No OSA (N=40, AHI < 5 /hour) or Mild OSA (N=199, 5 to <15 /hour) based on their screening polysomnogram. Scores on their Epworth Sleepiness Scale (ESS), Stanford Sleepiness Scale (SSS), Hamilton Rating Scale for Depression (HAM-D), Profile of Mood States (POMS) and Sleep Apnea Quality of Life Index (SAQLI) were compared between groups.
Results: There were no significant differences between the No OSA and Mild OSA groups on any of the 5 measures: ESS (No OSA, 9.8 + 3.5 vs Mild OSA, 10.6 + 4.3, p=0.26), SSS,(2.8 + 0.9 vs. 2.9 + 1.0, p=0.52), HAM-D (4.6 + 3.0 vs. 4.9 + 4.7, p=0.27), POMS (33.5 + 22.3 vs. 28.7 + 22.0, p=0.70), SAQLI (4.5 + 0.8 vs. 4.7 + 0.7, p=0.39).
Conclusion: Individuals with mild OSA in this cohort do not have worse sleepiness, mood or quality of life in comparison to those without OSA.
For accompanying editorial click here.
Abbreviations
AHI Apnea Hypopnea Index
APPLES Apnea Long-term Efficacy Study
BMI Body Mass Index
HAM-D Hamilton Rating Scale for Depression
IRB Institutional Review Board
ESS Epworth Sleepiness Scale
OSA Obstructive Sleep Apnea
PSG Polysomnogram
POMS Profile of Mood States
RDI Respiratory Disturbance Index
SAQLI Sleep Apnea Quality of Life Index
SSS Stanford Sleepiness Scale
WAIS Wechsler Adult Intelligence Scale
Introduction
Obstructive sleep apnea (OSA) is an important sleep related breathing disorder with prevalence rates between 3-17% in men and 3-9% in women (1,2). With the rising trend of obesity, it is becoming increasingly more common (2,3). In a number of longitudinal cohort studies, severe OSA is associated with an increased incidence of hypertension, cardiovascular disease and death (4-9). It also is adversely associated with a number of neurocognitive and behavioral outcomes including depression (10), sleepiness (11), and poor quality of life (12).
The most commonly used metric to classify severity of OSA is the apnea-hypopnea index (AHI) which is the number of apnea or hypopnea events per hour of sleep. Persons with an AHI < 5 are not considered to have OSA (13). In contrast, an AHI > 5 and < 15, AHI > 15 and <30, and an AHI > 30 are classified as mild, moderate, and severe respectively (14). It is generally accepted that OSA can negatively impact mood, wakefulness and quality of life. However, it is unclear whether mild OSA can have such effects (10, 11, 15). Epidemiological studies have generally shown that individuals with OSA are sleepier than those without OSA (16). Existing data in persons with mild OSA referred to sleep clinics are either limited primarily to assessments of sleepiness or have conflicting results (12, 17, 18).
The Apnea Positive Pressure Long-term Efficacy Study (APPLES) is a randomized, double-blinded, sham-controlled, multi-center trial of continuous positive airway pressure (CPAP) therapy designed to determine whether CPAP improves neurocognitive function over a 6-month test period (19). The present study is an analysis of the relationship between assessments of mood, sleepiness and quality of life in those without OSA versus mild OSA at the baseline visit (pre-randomization) in those screened for participation in APPLES. Our intent was to determine whether there was any association between mild OSA and these domains.
Methods
Participants and Study Design
The study design, recruitment procedures, and inclusion and exclusion criteria for APPLES have been described extensively (19). The institutional review board (IRB) at each site approved the study protocol. Briefly, APPLES was a multisite study conducted at 5 clinical centers: Stanford University, Stanford, CA; University of Arizona, Tucson, AZ; Providence St. Mary Medical Center, Walla Walla, WA; St. Luke’s Hospital, Chesterfield, MO; and Brigham and Women’s Hospital, Boston, MA. Participants were recruited into the study primarily from patients scheduled into a regular sleep clinic for evaluation of possible OSA, and from local advertising. Recruitment began in November 2003 and was completed in August 2008. Initial enrollment required age > 18 years and clinical symptoms of OSA, as defined by American Academy of Sleep Medicine (AASM) criteria (14). At enrollment, participants underwent a screening diagnostic polysomnogram (PSG) and baseline neurocognitive testing including the standardized assessments described below. Only participants with an apnea hypopnea index (AHI) > 10 events per hour continued to the clinical trial and were randomized subsequently to sham or active CPAP for 6 months as previously reported (19). Excluded were individuals who had 1) prior OSA treatment with CPAP or surgery, 2) household members with current/past CPAP use, 3) a sleepiness-related automobile accident within the year prior to potential enrollment, (4) oxygen saturations < 75% for > 10% of the diagnostic polysomnogram (PSG) total sleep time; or (5) conditions or use of medications that could potentially affect neurocognitive function and/or alertness. For the present analysis, data from both randomized and non randomized participants at the time of the screening polysomnography visit were utilized. In addition to new information, some of the material related to sleepiness reported herein represent reanalysis of data in a different format from what has been published in a previous paper (20).
Polysomnography
Polysomnography was conducted as previously described using signals from a nasal pressure cannula, nasal/oral thermistor, thoracic and abdominal piezo bands, and a pulse oximeter to classify apnea and hypopnea events. An apnea was identified by a > 90% amplitude decrease from baseline of the nasal pressure signal lasting > 10 sec. Hypopneas were scored if there was a > 50%, but < 90% decrease from baseline of the nasal pressure signal, or if there was a clear amplitude reduction of the nasal pressure signal that did not reach the above criterion but it was associated with either an oxygen desaturation > 3% or an arousal, and the event duration was ≥ 10 seconds. Obstructive apneas were identified by persistence of chest or abdominal respiratory effort during flow cessation. Central apneas were noted if no displacement occurred on either the thoracic or abdominal channels. All studies were scored at the central reading center located at Stanford University.
Assessments of Sleepiness
Epworth Sleepiness Scale (ESS): The ESS is a validated self-administered questionnaire that asks an individual to rate his or her probability of falling asleep on a scale of increasing probability from 0 to 3 in 8 different situations (21). The scores for the 8 questions are summed to obtain a single score from 0 to 24 that is indicative of self-reported sleep propensity. The ESS prior to randomization was administered at the time of the clinical evaluation and on the night of the diagnostic PSG. The value at the time of the diagnostic PSG was used, but if not available, then the value at the time of the clinical evaluation was substituted.
Stanford Sleepiness Scale (SSS): The SSS asks a person to rate current moment sleepiness on a scale of one to seven (22). Each numerical rating has an associated descriptor, for example a rating of 1 is described as “feeling active, vital, alert, or wide awake,” while a rating of 7 is described as “no longer fighting sleep, sleep onset soon; having dream-like thoughts.” For APPLES the SSS was administered at 10:00, 12:00, 14:00, and 16:00 on the day following the diagnostic PSG; the variable analyzed was the mean score from these 4 trials.
Assessments of Mood
Profile of Mood States (POMS): The POMS assesses mood by asking respondents how they feel at that moment according to a series of 65 descriptors such as “unhappy, tense or cheerful” (23). Possible responses are not at all, a little, moderately; quite a lot, extremely. Six mood states are used in the POMS: tension, depression, anger, vigor, fatigue, and confusion, which can be combined to form the total POMS mood disturbance score. Higher scores represent more negative mood states. For this analysis, total mood disturbance score was used.
Hamilton Rating Scale for Depression (HAM-D): The HAM-D is a validated 21-item clinician-administered assessment of the severity of depression (24). APPLES used a modified version of this test, the GRID Hamilton Rating Scale for Depression that was developed through a broad-based international consensus process to both simplify and standardize administration and scoring in clinical practice and research (25). In this scale, 17 items (e.g., depressed mood, suicide, work and anhedonia, retardation, agitation, gastrointestinal or general somatic symptoms, hypochondriasis, loss of insight or weight) are scored using either a 3- or 5-point scale based on intensity and frequency, and are summed to provide a single score. Higher scores reflect more depressive symptoms.
Quality of Life Assessment
Calgary Sleep Apnea Quality of Life Index (SAQLI): The SAQLI was developed as a sleep apnea specific quality of life instrument (26). It is a 35 item instrument that captures the adverse impact of sleep apnea on 4 domains: daily functioning, social interactions, emotional functioning and symptoms. Items are scored on a 7- point scale with “all of the time” and “not at all” being the most extreme responses. Item and domain scores are averaged to yield a composite total score between 1 and 7. Higher scores represent better quality of life.
Statistical Analyses
For this analysis, participants who had an AHI < 5 were assigned to the No OSA group, and those who had an AHI > 5, but < 15 were assigned to the Mild OSA group. Body mass index (BMI) was computed as weight (kg)/height (m)2. Participants’ race/ethnicity were classified as self-reported white or non-white. Marital status was categorized as married or not married. For continuous variables, unadjusted comparisons between the No OSA and Mild OSA groups were made using Student’s t-test. Differences in proportions were assessed using the χ2 test. Analysis of covariance was performed to adjust for differences in study site, age and BMI. Data are expressed as mean + standard deviation (SD) or percentages. P < 0.05 was considered statistically significant. Analyses were performed using IBM SPSS Statistics Version 20 (Chicago, IL).
Results
In Table 1 are shown the demographic data for the No OSA and Mild OSA groups.
Table 1: Demographic Information
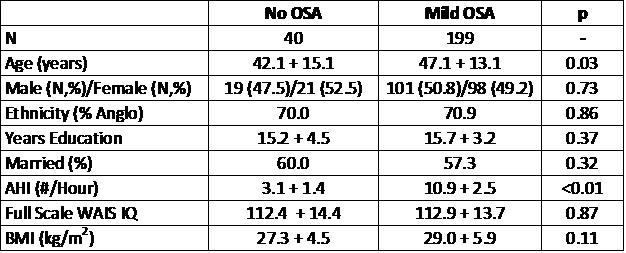
The groups were comparable with respect to gender, race, educational achievement, marital status and intelligence. By definition, the AHI for the Mild OSA group was significantly higher than for the No OSA group (10.9 + 2.5 vs. 3.1 + 1.4, p<0.01). However, participants in the No OSA were slightly younger than those in the Mild OSA group (42.1 + 15.1 vs. 47.1 + 13.1 years, p=0.03). There also was a slight trend for those in the Mild OSA group to have a higher BMI (27.3 + 4.5 vs. 29.0 + 5.9 kg/m2, p=0.11). Some differences related to study site were noted as well. For the HAM-D, there was a trend for the mean score of both groups combined to be higher at the Brigham and Women’s Hospital site [N=51] in comparison to the University of Arizona site [N=59] (6.1 + 5.3 vs. 3.6 + 3.6, p=0.046). Similarly, there was a trend for the ESS to be lower at the University of Arizona site [N=61] comparison to the St. Luke’s Hospital Site [N=29] (9.2 + 33 vs. 11.1 + 3.3, p=0.051).
Table 2 shows the comparisons between the No OSA and Mild OSA groups for the sleepiness, mood and quality of life metrics.
Table 2: Sleepiness, Mood and Quality of Life in No OSA and OSA Groups

There were no statistically significant differences observed for any of these variables. The table also shows the power in this study to detect clinically significant differences in these metrics. As shown, there is 90% power to demonstrate a 1.92, 0.52, 12.72, 1.90 and 0.45 difference between groups in the ESS, SSS, POMS, HAM-D and SAQLI respectively. Furthermore, although the No OSA group was slightly younger, there were no significant correlations between age and the ESS, SSS, POMS, HAM-D, and the SAQLI (r values between 0.04 and 0.11).
Discussion
In this analysis, we show that using a commonly accepted definition of mild OSA, sleepiness and mood are not different in comparison to persons without significant OSA. Furthermore, there was no evidence that mild OSA negatively impacts quality of life. These data suggest that mild OSA as currently defined has little adverse impact on sleepiness, mood and quality of life.
We observed that there were no differences in the ESS between participants with No OSA in comparison to those with Mild OSA. Results from other large cohorts are conflicting. Our results are consistent with those of Lopes et al (12) who also did not find that the ESS was elevated in those with Mild OSA in a large population of patients undergoing PSG for suspected OSA. In contrast, a cohort of Chinese patients with mild OSA had a greater prevalence of subjective daytime sleepiness in comparison to those with primary snoring (18). However, the ESS was not higher in contrast to the Sleep Heart Health Study in which the ESS appeared to be greater in those with Mild OSA (16). Similarly, excessive daytime sleepiness was more commonly reported among a cohort of Japanese women participating in a cardiovascular risk study (17). In this latter study, OSA status was determined using pulse oximetry and not PSG. A number of other studies also have reported sleepiness data in subjects with mild OSA. However, small sample sizes, populations with specialized characteristics, and lack of specific comparisons between persons with mild OSA and no OSA limit their interpretability (27-32).
In this study, mood as assessed by the POMS and the HAM-D was not worse in the Mild OSA group. Although depressive symptoms and use of anti-depressants are commonly noted among patients with OSA (33-35), studies of whether mood is affected by mild OSA are few. In 2 studies performed in patients seen in an otolaryngology clinic (27, 31), the Beck's Depression Inventory (BDI) was not different in comparison to either a control group or primary snorers. Similarly, in a group of elderly Koreans referred to a sleep clinic, the BDI was not elevated in comparison to an age-matched control group (36). Our findings extend these previous reports by showing that using two different assessments of mood, there was no adverse impact of mild OSA.
Quality of life in this study was not affected by mild OSA. In contrast, in a number of studies, quality of life assessed with various instruments is impaired in persons with OSA (37-40). However, there are few studies in which the potential impact of mild OSA has been examined. In a relatively small study performed in patients from an otolaryngology clinic, scores on the SAQLI in patients with mild OSA were the same as a group of primary snorers (31). Similarly, in an analysis of 461 elderly women who underwent PSG in the Study of Osteoporotic Fractures cohort, scores on the Functional Outcomes of Sleep Questionnaire were the same across tertiles of OSA severity (41). Thus, our findings demonstrating a lack of association between mild OSA and quality of life are consistent with these previous studies.
Our failure to demonstrate an association between mild OSA and sleepiness, mood and quality of life provides additional data challenging the commonly used threshold for “defining disease” in the assessment of OSA. The traditional cutpoint of 5 originated more than 30 years ago when only apneic events were scored (42, 43). In the intervening years, it has been accepted that hypopneas have pathophysiologic significance and are now incorporated into the AHI (44). Additionally, some clinicians advocate including the more subtle respiratory effort related arousals into a broader respiratory disturbance index (RDI) (45). The data in this study suggest that at least for some domains of OSA symptomatology, mild OSA based on the application of current scoring criteria to older thresholds may in fact be part of a normal population.
Despite our findings, clinicians, insurers and policy makers should be cautioned about using the AHI as the sole metric in determining whether or not to treat an individual patient. The impact of OSA insofar as behavioral and neurocognitive domains are concerned appears to be quite heterogeneous. For example, 54% of individuals in the Sleep Heart Health Study with moderate to severe OSA were not sleepy on any one of 3 measures of sleepiness. Conversely, some individuals with less severe OSA may be sleepy (16). In our study, the mean ESS in both the No OSA and Mild OSA groups was above what would be expected for an unselected general population suggesting that other causes of sleepiness were present in the cohort (16). Thus, before deciding to initiate OSA specific treatment for Mild OSA, clinicians should consider whether there are other explanations for the patient’s symptoms, and not just treat the AHI.
This study does have three major limitations. First, it might be argued that our study was underpowered to detect small differences between the No OSA and Mild OSA groups. However, sufficient statistical power was present to detect clinically important differences (Table 2). For example, it has been proposed that the minimally important difference on repeated administrations of the SAQLI is approximately 1 (46). Our results demonstrated that we had 90% power to detect a change of 0.5. Moreover, our findings are consistent with the limited number of studies previously performed. Second, our participants were a mixture of individuals recruited from sleep clinics and those responding to advertisements. Thus, they may not be representative of the general populace. Third, it is possible that the No OSA group included some individuals who actually had mild OSA. Inasmuch as all participants were considered by clinicians to have symptoms consistent with OSA, some individuals in the No OSA group may have had falsely “negative” PSGs. Such misclassification would bias towards a null effect. The extent to which this occurred is not known, but night to night variability of the AHI is relatively low (47). Thus, we suspect this potential bias is small. Despite these limitations, however, the APPLES cohort was geographically and ethnically diverse, and had a representative gender distribution.
In conclusion, evidence from this analysis does not indicate that mild OSA has any impact on sleepiness, mood or quality of life. This raises concerns whether the current AHI criteria for distinguishing mild OSA from no clinically significant OSA needs to be reassessed. Nevertheless, additional comparisons between individuals who are truly without OSA symptoms and those with mild OSA as currently defined need to be performed before a final conclusion can be determined.
References
- Punjabi NM. The epidemiology of adult obstructive sleep apnea. Proc Am Thorac Soc. 2008;5(2):136-143. [CrossRef] [PubMed]
- Peppard PE, Young T, Barnet JH, Palta M, Hagen EW, Hla KM. Increased prevalence of sleep-disordered breathing in adults. Am J Epidemiol. 2013;177(9):1006-1014. [CrossRef] [PubMed]
- Inge TH, King WC, Jenkins TM, et al. The effect of obesity in adolescence on adult health status. Pediatrics. 2013;132(6):1098-1104. [CrossRef] [PubMed]
- Peppard PE, Young T, Palta M, Skatrud J. Prospective study of the association between sleep-disordered breathing and hypertension. N Engl J Med. 2000;342(19):1378-84. [CrossRef] [PubMed]
- Young T, Finn L, Peppard PE, et al. Sleep disordered breathing and mortality: eighteen-year follow-up of the Wisconsin sleep cohort. Sleep. 2008;31(8):1071-1078. [PubMed]
- Gottlieb DJ, Yenokyan G, Newman AB, et al. Prospective study of obstructive sleep apnea and incident coronary heart disease and heart failure: the sleep heart health study. Circulation. 2010;122(4):352-360. [CrossRef] [PubMed]
- Marshall NS, Wong KK, Liu PY, Cullen SR, Knuiman MW, Grunstein RR. Sleep apnea as an independent risk factor for all-cause mortality: the Busselton Health Study. Sleep. 2008;31(8):1079-1085. [PubMed]
- Punjabi NM, Caffo BS, Goodwin JL, et al. Sleep-disordered breathing and mortality: a prospective cohort study. PLoS Med . 2009;6(8):e1000132. [CrossRef] [PubMed]
- Marin JM, Carrizo SJ, Vicente E, Agusti AG. Long-term cardiovascular outcomes in men with obstructive sleep apnoea-hypopnoea with or without treatment with continuous positive airway pressure: an observational study. Lancet. 2005;365(9464):1046-1053. [CrossRef] [PubMed]
- Schroder CM, O'Hara R. Depression and Obstructive Sleep Apnea (OSA). Ann Gen Psychiatry. 2005;4:13. [PubMed]
- Jordan AS, McSharry DG, Malhotra A. Adult obstructive sleep apnoea. Lancet. 2014;383(9918):736-747. [CrossRef] [PubMed]
- Lopes C, Esteves AM, Bittencourt LRA, Tufik S, Mello MT. Relationship between the quallity of life and the severity of obstructive sleep apnea syndrome. Braz J Med Biol Res. 2008;41(10):908-913. [CrossRef] [PubMed]
- Sateia MJ. International Classification of Sleep Disorders 2nd ed. Westchester, IL: American Academy of Sleep Medicine, 2005; 297.
- American Academy of Sleep Medicine Taskforce. Sleep-Related Breathing Disorders In Adults: Recommendations For Syndrome Definition And Measurement Techniques In Clinical Research. Sleep. 1999;22 (5): 667-689. [PubMed]
- Ayas NT, Hirsch AA, Laher I, et al. New frontiers in obstructive sleep apnoea. Clin Sci (Lond). 2014;127(4):209-216. [CrossRef] [PubMed]c
- Gottlieb DJ, Whitney CW, Bonekat WH, et al. Relation of sleepiness to respiratory disturbance index: the Sleep Heart Health Study. Am J Respir Crit Care Med . 1999;159(2):502-507. [CrossRef] [PubMed]
- Cui R, Tanigawa T, Sakurai S, et al. Associations of sleep-disordered breathing with excessive daytime sleepiness and blood pressure in Japanese women. Hypertens Res. 2008;31(3):501-506. [CrossRef] [PubMed]
- Chen R, Xiong KP, Lian YX, et al. Daytime sleepiness and its determining factors in Chinese obstructive sleep apnea patients. Sleep Breath. 2011;15(1):129-135. [CrossRef] [PubMed]
- Kushida CA, Nichols DA, Quan SF, et al. The Apnea Positive Pressure Long-term Efficacy Study (APPLES): rationale, design, methods, and procedures. J Clin Sleep Med. 2006;2(3):288-300. [PubMed]
- Quan SF, Chan CS, Dement WC, et al. The association between obstructive sleep apnea and neurocognitive performance--the Apnea Positive Pressure Long-term Efficacy Study (APPLES). Sleep. 2011;34(3):303-314B. [PubMed]
- Johns MW. A new method for measuring daytime sleepiness: the Epworth sleepiness scale. Sleep. 1991;14(6):540-545. [PubMed]
- Hoddes E, Dement W, Zarcone V. The development and use of the Stanford Sleepiness Scale (SSS). Psychophysiol. 1972;9:150.
- McNair DM, Lorr M, Droppleman L. Manual for the Profile of Mood States. San Diego, CA: Educational and Industrial Testing Service, 1971;
- Hamilton M. A rating scale for depression. J Neurol Neurosurg Psychiatry. 1960;2356-62.
- Williams JB, Kobak KA, Bech P, et al. The GRID-HAMD: standardization of the Hamilton Depression Rating Scale. Int Clin Psychopharmacol. 2008;23(3):120-129. [CrossRef] [PubMed]
- Flemons WW, Reimer MA. Development of a disease-specific health-related quality of life questionnaire for sleep apnea. Am J Respir Crit Care Med. 1998;158(2):494-503. [CrossRef] [PubMed]
- Ishman SL, Cavey RM, Mettel TL, Gourin CG. Depression, sleepiness, and disease severity in patients with obstructive sleep apnea. Laryngoscope. 2010;120(11):2331-2335. [CrossRef] [PubMed]
- Minoguchi K, Yokoe T, Tazaki T, et al. Silent brain infarction and platelet activation in obstructive sleep apnea. Am J Respir Crit Care Med. 2007;175(6):612-617. [CrossRef] [PubMed]
- Yoshino A, Higuchi M, Kawana F, et al. Risk factors for traffic accidents in patients with obstructive sleep apnea syndrome. Sleep Biol Rhythms. 2006;4144-152.
- Back L, Palomaki M, Piilonen A, Ylikoski J. Sleep-disordered breathing: radiofrequency thermal ablation is a promising new treatment possibility. Laryngoscope. 2001;111(3):464-471. [CrossRef] [PubMed]
- Balsevicius T, Uloza V, Sakalauskas R, Miliauskas S. Peculiarities of clinical profile of snoring and mild to moderate obstructive sleep apnea-hypopnea syndrome patients. Sleep Breath. 2012;16(3):835-843. [CrossRef] [PubMed]
- Lecube A, Sampol G, Lloberes P, et al. Asymptomatic sleep-disordered breathing in premenopausal women awaiting bariatric surgery. Obes Surg. 2010;20(4):454-461. [CrossRef] [PubMed]
- Saunamaki T, Jehkonen M. Depression and anxiety in obstructive sleep apnea syndrome: a review. Acta Neurol Scand. 2007;116(5):277-288. [CrossRef] [PubMed]
- Ohayon MM. The effects of breathing-related sleep disorders on mood disturbances in the general population. J Clin Psychiatry. 2003;64(10):1195-200; quiz, 1274-6. [CrossRef] [PubMed]
- Chandra RK, Epstein VA, Fishman AJ. Prevalence of depression and antidepressant use in an otolaryngology patient population. Otolaryngol Head Neck Surg. 2009;141(1):136-138. [CrossRef] [PubMed]
- Ju G, Yoon IY, Lee SD, Kim TH, Choe JY, Kim KW. Effects of sleep apnea syndrome on delayed memory and executive function in elderly adults. J Am Geriatr Soc. 2012;60(6):1099-1103. [CrossRef] [PubMed]
- Baldwin CM, Ervin AM, Mays MZ, et al. Sleep disturbances, quality of life, and ethnicity: the Sleep Heart Health Study. J Clin Sleep Med. 2010;6(2):176-183. [PubMed]
- Baldwin CM, Griffith KA, Nieto FJ, O'Connor GT, Walsleben JA, Redline S. The association of sleep-disordered breathing and sleep symptoms with quality of life in the Sleep Heart Health Study. Sleep. 2001;24(1):96-105.[PubMed]
- Isidoro SI, Salvaggio A, Bue AL, Romano S, Marrone O, Insalaco G. Quality of life in patients at first time visit for sleep disorders of breathing at a sleep centre. Health Qual Life Outcomes. 2013;11207.
- Stepnowsky C, Johnson S, Dimsdale J, Ancoli-Israel S. Sleep apnea and health-related quality of life in African-American elderly. Ann Behav Med. 2000;22(2):116-120. [CrossRef] [PubMed]
- Kezirian EJ, Harrison SL, Ancoli-Israel S, et al. Behavioral correlates of sleep-disordered breathing in older women. Sleep. 2007;30(9):1181-1188. [PubMed]
- Guilleminault C. Sleep and Breathing. In: Sleeping and Waking Disorders: Indications and Techiniques. Guilleminault C, ed. Menlo Park, CA: Addison-Wesley, 1982; 155-182
- Block AJ, Boysen PG, Wynne JW, Hunt LA. Sleep apnea, hypopnea and oxygen desaturation in normal subjects. N Engl J Med. 1979;300 (10):513-517. [CrossRef] [PubMed]
- Gould GA, Whyte KF, Rhind GB, et al. The sleep hypopnea syndrome. Am Rev Respir Dis .1988;137(4):895-898. [CrossRef] [PubMed]
- Pepin JL, Guillot M, Tamisier R, Levy P. The upper airway resistance syndrome. Respiration. 2012;83(6):559-566. [CrossRef] [PubMed]
- Flemons WW, Reimer MA. Measurement properties of the calgary sleep apnea quality of life index. Am J Respir Crit Care Med. 2002;165(2):159-164. [CrossRef]
- Quan SF, Griswold ME, Iber C, et al. Short-term variability of respiration and sleep during unattended nonlaboratory polysomnography--the Sleep Heart Health Study. Sleep. 2002;25(8):843-849. [PubMed]
Acknowledgements
APPLES was funded by contract 5UO1-HL-068060 from the National Heart, Lung and Blood Institute. The APPLES pilot studies were supported by grants from the American Academy of Sleep Medicine and the Sleep Medicine Education and Research Foundation to Stanford University and by the National Institute of Neurological Disorders and Stroke (N44-NS-002394) to SAM Technology. In addition, APPLES investigators gratefully recognize the vital input and support of Dr. Sylvan Green who died before the results of this trial were analyzed, but was instrumental in its design and conduct.
Administrative Core
Clete A. Kushida, MD, PhD; Deborah A. Nichols, MS; Eileen B. Leary, BA, RPSGT; Pamela R. Hyde, MA; Tyson H. Holmes, PhD; Daniel A. Bloch, PhD; William C. Dement, MD, PhD
Data Coordinating Center
Daniel A. Bloch, PhD; Tyson H. Holmes, PhD; Deborah A. Nichols, MS; Rik Jadrnicek, Microflow, Ric Miller, Microflow Usman Aijaz, MS; Aamir Farooq, PhD; Darryl Thomander, PhD; Chia-Yu Cardell, RPSGT; Emily Kees, Michael E. Sorel, MPH; Oscar Carrillo, RPSGT; Tami Crabtree, MS; Booil Jo, PhD; Ray Balise, PhD; Tracy Kuo, PhD
Clinical Coordinating Center
Clete A. Kushida, MD, PhD, William C. Dement, MD, PhD, Pamela R. Hyde, MA, Rhonda M. Wong, BA, Pete Silva, Max Hirshkowitz, PhD, Alan Gevins, DSc, Gary Kay, PhD, Linda K. McEvoy, PhD, Cynthia S. Chan, BS, Sylvan Green, MD
Clinical Centers
Stanford University
Christian Guilleminault, MD; Eileen B. Leary, BA, RPSGT; David Claman, MD; Stephen Brooks, MD; Julianne Blythe, PA-C, RPSGT; Jennifer Blair, BA; Pam Simi, Ronelle Broussard, BA; Emily Greenberg, MPH; Bethany Franklin, MS; Amirah Khouzam, MA; Sanjana Behari Black, BS, RPSGT; Viola Arias, RPSGT; Romelyn Delos Santos, BS; Tara Tanaka, PhD
University of Arizona
Stuart F. Quan, MD; James L. Goodwin, PhD; Wei Shen, MD; Phillip Eichling, MD; Rohit Budhiraja, MD; Charles Wynstra, MBA; Cathy Ward, Colleen Dunn, BS; Terry Smith, BS; Dane Holderman, Michael Robinson, BS; Osmara Molina, BS; Aaron Ostrovsky, Jesus Wences, Sean Priefert, Julia Rogers, BS; Megan Ruiter, BS; Leslie Crosby, BS, RN
St. Mary Medical Center
Richard D. Simon Jr., MD; Kevin Hurlburt, RPSGT; Michael Bernstein, MD; Timothy Davidson, MD; Jeannine Orock-Takele, RPSGT; Shelly Rubin, MA; Phillip Smith, RPSGT; Erica Roth, RPSGT; Julie Flaa, RPSGT; Jennifer Blair, BA; Jennifer Schwartz, BA; Anna Simon, BA; Amber Randall, BA
St. Luke’s Hospital
James K. Walsh, PhD, Paula K. Schweitzer, PhD, Anup Katyal, MD, Rhody Eisenstein, MD, Stephen Feren, MD, Nancy Cline, Dena Robertson, RN, Sheri Compton, RN, Susan Greene, Kara Griffin, MS, Janine Hall, PhD
Brigham and Women’s Hospital
Daniel J. Gottlieb, MD, MPH, David P. White, MD, Denise Clarke, BSc, RPSGT, Kevin Moore, BA, Grace Brown, BA, Paige Hardy, MS, Kerry Eudy, PhD, Lawrence Epstein, MD, Sanjay Patel, MD
*Sleep HealthCenterscfor the use of their clinical facilities to conduct this research
Consultant Teams
Methodology Team: Daniel A. Bloch, PhD, Sylvan Green, MD, Tyson H. Holmes, PhD, Maurice M. Ohayon, MD, DSc, David White, MD, Terry Young, PhD
Sleep-Disordered Breathing Protocol Team: Christian Guilleminault, MD, Stuart Quan, MD, David White, MD
EEG/Neurocognitive Function Team: Jed Black, MD, Alan Gevins, DSc, Max Hirshkowitz, PhD, Gary Kay, PhD, Tracy Kuo, PhD
Mood and Sleepiness Assessment Team: Ruth Benca, MD, PhD, William C. Dement, MD, PhD, Karl Doghramji, MD, Tracy Kuo, PhD, James K. Walsh, PhD
Quality of Life Assessment Team: W. Ward Flemons, MD, Robert M. Kaplan, PhD
APPLES Secondary Analysis-Neurocognitive (ASA-NC) Team: Dean Beebe, PhD, Robert Heaton, PhD, Joel Kramer, PsyD, Ronald Lazar, PhD, David Loewenstein, PhD, Frederick Schmitt, PhD
National Heart, Lung, and Blood Institute (NHLBI)
Michael J. Twery, PhD, Gail G. Weinmann, MD, Colin O. Wu, PhD
Data and Safety Monitoring Board (DSMB)
Seven year term: Richard J. Martin, MD (Chair), David F. Dinges, PhD, Charles F. Emery, PhD, Susan M. Harding MD, John M. Lachin, ScD, Phyllis C. Zee, MD, PhD
Other term: Xihong Lin, PhD (2 yrs), Thomas H. Murray, PhD (1 yr).
None of the authors claim any conflicts of interest relevant to the article.
Reference as: Quan SF, Budhiraja R, Batool-Anwar S, Gottlieb DJ, Eichling P, Patel S, Shen W, Walsh JK, Kushida CA. Lack of impact of mild obstructive sleep apnea on sleepiness, mood and quality of life. Southwest J Pulm Crit Care. 2014;9(1):44-56. doi: http://dx.doi.org/10.13175/swjpcc082-14 PDF